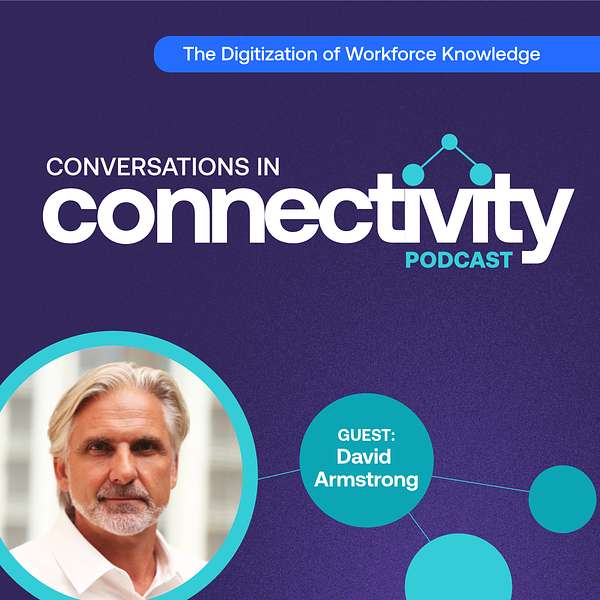
What to Expect When You're Connecting
What to Expect When You're Connecting includes interviews with a wide range of industry subject matter experts who share their journey, advice, and the mistakes they've made along the way in IoT. If you're adding connectivity to your products for the first time or seeking to optimize and scale your existing connectivity operations – welcome to the conversation.
What to Expect When You're Connecting
The Digitalization of Workforce Knowledge with David Armstrong
Digitalization is an incredibly dense topic, especially when we’re talking about the digitalization of knowledge within a workforce. Not as a way to replace people, but to preserve the knowledge that allows us to trust the feedback systems (whether it’s analog or digital) and bring confidence back into our decision-making.
Connectivity, data collection, and sensors are only as good as the underlying data models, assumptions, and the data science we apply to a solution … to which our guest said during the panel, “you know, long ago we had another name for data science, it was called math”
So what does this conversation have to do with connectivity? Everything.
Digitization goes well beyond individual discrete data points and it’s clear that the data we need to make meaningful decisions doesn’t stop at the four walls of a facility — it requires pulling data from “out there”.
Welcome to conversations and conductivity. I'm Ryan Carlson, your host. And within each episode, you'll find curated conversations from all corners of conductivity, the internet of things, smart products, and the industries that are building and deploying these technologies to solve problems all around us. This episode is brought to you by Soracom, a global connectivity service provider that believes the fastest way to cost savings and scale is when customers are in full control of their connectivity operations. You can sign up for an operator account to experience self Pays you go global conductivity without a contract today at soracom.io today's guest is David Armstrong, a digitalization evangelist, and a director at a company called cohesive. Now we had the pleasure of sitting down with him after his participation in a panel discussion. About the economic environmental value of digital transformation projects, which was at the IOT in oil and gas show of Houston, Texas. Okay, who nailed it? You'll understand in a moment. have you ever sat through a panel discussion? You know, one of those ones where you just keep hoping that the microphone gets handed back to a particular panelist, because everything they're saying about a topic just kind of cuts right through the noise. Well, digitization or digitalization is an incredibly dense topic. especially when we're talking about the digitization or digitalization. Of knowledge within a workforce not as a way to replace people, but to preserve the knowledge that allows us to trust the feedback systems, whether it's analog or digital and bring confidence back into our decision-making. Conductivity data collection and sensors. Now these are all as good. Or only as good as the underlying data models, assumptions and the data science that we apply to a solution. Now, this is something to which our guests said during the panel, he was in, you know, long ago we had another name for data science. It was called math. What does this conversation have to do with connectivity? Kind of everything. Because digitization. He goes well beyond individual discrete data points. And it's clear that the data that we're going to need in order to make meaningful decisions. Beyond just discrete alarms and alerts it doesn't stop at the four walls of a facility. It requires pulling data from out there so without further delay let's get on with the interview
Ryan:So David we're at the oil oil T I'm gonna start over on that one We're at the oil T I'm gonna say that over and over again right
David:T conference for oil and gas
Ryan:Thank you so much
David:the energy conference network
Ryan:You today You've got the whole thing down How much are they paying you
David:Yeah On I get lunch
Ryan:I got is that why I got lunch too
David:you what You get lunch the same way
Ryan:So you said something really fascinating in the panel discussion the other day in which you were talking about the need to digitize the knowledge of a workforce That is slowly exiting either because they wanna retire or they're dying
David:That's right. No it's not as sexy to be in a trade And to you to apply your to to use your To recognize the environment that you're working with and understand holistically how an asset changes and to pull the individual levers that might affect that positively or negatively And I think that's a missing skill I'm a big believer that uh you know we've got white collar jobs we've got blue collar jobs and I think those are gold collar jobs I today they're getting paid a substantial amount more and maybe they're not getting paid enough so they should be gold collar because that could be the missing jewel that we're gonna achieve Digitalization
Ryan:So when you hear about digitizing a workforce what does that mean to someone who's doing a lot of big stuff with data
David:So I think fundamentally we have to look at and compare what the IOT space can deliver Right I mean how much of the asset base can actually be digitalized and automated So we're receiving signals and what are those So I always say that we're getting effect level data right So we're getting a signal that says there's a problem but what actually caused that problem it's usually a many to one relationship Okay And there are processes that have been they're well defined They're well known Anything from RCM to has off to CIS SI Fundamentally what they do is they sort out what the are the discrete data points that contribute to cause and event whether it be vibration high temperature flows going outta whack or why is the bus back tires wobbling it was it's many different reasons could be the road could be the fact that the bearings are shot could be the fact that the bus is overloaded That's what IoT can bring but the trades people that preceded IoT used to have to be able to deduce that information themselves Using the six senses So we know about the six senses but then there's also the other senses where the human brain where we use what like one to 2% maybe some Some that are special like yourself use 3% but I that's not a good return
Ryan:That's 33% more than average I'll take that gotta use the right
David:but I mean so let's build on that so inside our brains we can recognize that there are multiple factors that cause that event that's the stuff that we need to digitalize cuz that's to me that's the missing ingredient that's not the 30 let's say IOT can deliver 30 to 40% I'm gonna be a little generous there Cause I don't think it's even that high It's that bigger piece that once we've digitalized that then we can leverage AI and machine learning and blockchain and whatever to drill down
Ryan:most of what we're dealing with aren't technology problems they're people problems So what I've seen is people using technology for example there was a large steel plant that creates the trucks for trains and they took a bunch of their guys who are near retirement age and they put'em in SOC So they get a sit back and they've got brand new people who are going out They've got wireless webcams And as you said they know the 27 different right questions to ask to narrow down to the what the problem is Whereas the sensors have no idea They're all just going weep weep All right Check here Check this weep
David:gonna expand I think some of the sensors are getting smarter like vibration they can see when a signal is leading to something but
Ryan:sure With the motor vibration analysis And but that that that's a good machine learning though Getting establish the baseline looking for variations but what are we doing for that institutional knowledge that the person in the so center has I'm really curious to hear what does that look like Is it just having them train models
David:I think I don't think it's the training of models is the validation of the models in a deterministic way or actually accurate and making sense so I think we need to I hate to use the term unpacked that a little bit cuz it's so
MIC1:yeah it's pop the hood Let's go
David:Yeah But when I think a deterministic model a rule based model is the first step in digitalizing what the tradesperson sees And if I talk about those wheels on the bus or wheels on the back of the rail car on the bogie What actually contributed to that vibration issue was it the track Was it the wheel was it a source of lubrication Was it the wrong bearing size Maybe somebody pressed a bearing in and the tolerance was too tight That's those are the stories that are getting lost So those are the stories that getting lost not what is happening but why it happened And the why it happened is really important because when organizations understand why They can put strategies to accept manage mitigate or model remodel and put solutions around that risk That's where organizations move from Good to great When an organization goes ah on that bearing race it wasn't a lubrication issue It It manifested into vibration issue but somebody took a bearing off a store shelf that was perhaps dropped or it was or that when they pressed the race in on the bearing the tolerance was too tight how do we solve that in the future So maybe we remachine it or maybe we prep it before we do it And We eliminate that from the potential problems moving forward and what you're doing Is you're actually eliminating all this many sources of variance So you can focus on what you can or need to actually manage And then you can accelerate your performance organization right You're reducing all the sources of variation
Ryan:So is it this is my personal belief in that human judgment will be the one thing that computers and AI is really can't eliminate but what does that line look like? Realistically if trying to solve really big problems is hard, So solving small discrete one discrete problem at a time You're talking about wheel bearings you told me a story about optimizing steel right Just how much are we getting for how many people do we need And actually no one else has actually heard that Would you mind sharing Like I thought that was a good example of tying the need to know things to business drivers that are meaningful to people who don't care what actually happened
David:So in the example we talked about steel company needed to figure out how they could get more yield more return from the steel that they were rolling So in their hots strip mills yield losses happen when you cut the end of the coil off or how much goes into the pit at the end the trash pit and then they gotta put it back in the fiery furnace Or but year losses can also happen by running too thicker material or too thinner material Too slow So what controls that are actually load cells that give feedback back into your H HMI system or your operator control center to use a more generic term that the people want to hear about but so by understanding those controls we can understand if we're running at optimum speed optimum thickness optimum quality optimum return the issue is we need to be able to trust in those feedback systems So when we started to see dither in those feedback systems we can say aha right My confidence The decision making that I have to deliver here is being reduced I need to proactively change those at the right moment so I can get ahead of that So I can bring confidence back in my decision making
MIC1:Yep
David:you made point of the fact that I said the data science Before that it was called lean SIG Sigma before that it was called math When I took it I had a an economics professor in year one and university and she was an Aussie and she called it sad statistics which is statistics But it's the same strike It's just understanding where spreads and variances is happening and reducing those outliers So you're in a normal operating condition It's not hard It's just maybe it is hard but it's just we need to simplify right one bite it or the elephant We gotta chop the elephant down understand where the variance is coming from Understand what's caused it Is it real or is are my signaling systems in this case they're starting to They need recalibrating or they need changing out proactively So then I can go back and eliminate that source of noise validate what's going on and then continue to make oodles of money
Ryan:And so I'm hearing that it's not just understanding knowing where that dither is then it's operationalizing the We know that on average every six months we add this to our OEE checklist or we add this to our maintenance team we see this but it goes the people like how does this knowing
David:I'm gonna change that
MIC1:for second Okay go ahead
David:you did the you brought time into this So time is the fourth dimension right
MIC1:Whoa
David:four D who do we enter the Twilight zone time is something that everybody wants to predict on The issue is humans put humans of the fly in the ointment right So if we only check something every six months is that optimal Or should we be continually simp monitoring thing because they're super critical for us achieving our goals
MIC1:because we are mortal and entropy affects us differently than our machine overloads the things that we made Using other factors outside of time what is it that a machine can detect that we as humans can't that gives us the
David:machine should tell When to fix something not time or just because we've run a work order and it spits it out every six months and I'm sending three guys over there and two and two others to go and fix this machine because it's time that's just wrong Right
Ryan:I have been thinking about this so wrong for so long The fact that time should be the one thing that we eliminate in all of our conversations or at least be critical of it Are we using time Because it Us meet acks have a like limited scalability Like we can only drive
David:to when it's a box ticking exercise usually right Yes I've done my check It's it is yes doctor I've done my check your prognosis is I need to come back six months from now And the reality is that doesn't work work
Ryan:Time is the artificial constraint It's other data points that a true specialist in the Would be able to look at oh it's leaning a little bit to the left or hear that squeak yet Like exactly they've They've got I wish I could remember all of these great anecdotal things where you go out and you do qualitative data research and you have okay who's the most senior person here Tell me about why did you get a phone call in the middle of the
MIC1:What went wrong and what was probably the cause and you get some really great stories it's the guy who goes oh you just gotta take this balling hammer and hit it right here And
David:I've seen that
MIC1:So have I it's with car
David:guys with the the screwdriver to the side of the bearing Yeah and you know what that's all valid stuff And this is exact goes right back to what we discussed I can put an IOT sensor on something and spend thousands and put monitoring systems but maybe it's enough for the guy with the screw driver Qualified to put his ear to a screwdriver on the side of a gearbox and go you know what I'm getting a grinding noise every third revolution I know that something's going on with that second pinion gear or my lubrication is not right So then the challenge is once I collect that tacit information is how do I make you or me or that person over there hear the same noise in the same way. So what we're doing now is defining a discrete data point and then creating a repeatable reproducible data set where somebody completely foreign to the analysis can go oh it's box a Because that that click that they've heard is every third revolution or it's box B cuz that click is click click click click click click So those repeatable reproducible states are exactly how optometrists actually make
MIC1:a or
David:click or it's box C cuz the thing's on Right.
MIC1:C or D
David:Yeah It's psychometric scaling is what it is
Ryan:they do it for bike fitting too our instrumentation as humans It's really bad because we're getting all the senses in all at once We can't just say turn off everything but you're hearing Yeah But a machine can
David:And we're all calibrated differently And that's part of the issue How do we calibrate a human right to see things in the same So now it's a little bit different How do we calibrate our models to seed things the way a human would first we gotta define what we're looking at and then the states by what good looks like
Ryan:And this is why training programs are so hard because people learn differently They interpret stimulus differently So assuming that you can't just take the David model and say you are the best at this like trying to teach other people to do it the same way you do is hard to repeat And if even if you could it might A lot of time investment and scale. My question for you then is if we know that it's good enough to apply a lot of the same diagnostics those discre data points to it the very least just say you know what 88% likelihood there's a problem Send someone in is enough to intervene when do we say enough is enough to allow humans To minimize the level of effort and work up to their certification stop them from doing some of these menial things
David:There's a few things that are in my head from that when do we take the human out of the equation type or what's the right application
MIC1:I like to think of it as when do we let the human work on something more important
David:Yeah So a lot of the stuff that are incredibly valuable gold call trades people are looking at are things that they shouldn't be right We should be allowing IOT to to use to deliver a better service and constant monitoring those kind of things come into play there we can gain true condition out of it and not send a human to a place where they'd be better served doing something far more complex or rebuilding something because let's and let's talk about those trades people they're hard to find And the good ones are even harder to find and they're expensive So that's a better use of their time
Ryan:So we've identified the audience we know all of the people that would like to see improvements We know that trades people would like to be working up to their level of skill and not be doing menial tasks So we've got the audience We've got the problem Tell me a little bit more about what it You are doing that Uniquely addresses this audience and problem
David:So let's talk about those tradesmen for a second Let's say they've got these models in their head and they're able to look at 3, 5, 6 different potential anomalies That would cause that problem And that but they're very they're looking extremely myopically at one asset Okay Now another trades person who's potentially not on that same Knows a whole lot more about the asset beside it either in front of the process or behind the process Okay Now what we're able to do is sent by digitizing all that information right By capturing by harnessing that information Now we're able to apply a whole load of tradespeople to that one inspection point and understand all the relatable attributes that could be combined from a whole plethora of assets at the exact same time By using things like AI and machine learning because now we're opening up to the entire population of data that could have influenced that that asset from starting to fail
MIC1:So this is the crash reconstruction team that is looking at everything along a timeline with the strings
David:and maybe it's not within our systems maybe it was the impact of weather and things like Now we're talking about the whole population of data of IOT devices those billion trillion connections which obviously they don't all lie within our own organization but maybe they're things that happened weather changes humidity changes an uprising in the middle of somewhere And it caused a reduction in electricity in the grid and that caused the equipment into Right now by digitalizing all that and being able to create an inference against that broader set of population Now we can get highly confident models on why that event actually happened Not just solely reliant on that one trades person's knowledge Now it's all the potential trades persons knowledge in the organization or even better If we were to connect all our All the tradespeople in the world and all the data systems that we've connected to Now we can understand what part of the equation they all played in that event happening It
MIC1:you say we a lot Tell me a little bit more about the we
David:Yeah We as a big thing So in
MIC1:The Royal wheat
David:we it's the Royal we so we as in my organization we help digitalize that stuff We help connect all those systems in an object model architecture if we're gonna start to think about fixing things which are really hard like the impact of what humans have done to this earth we need to move to the Royal We it's really essential to understand what is contributing to how we're destroying the world What's contributing to how political events unfold and how One little flag that went up right Contributed to two flags that went up and all of a sudden we've got an uprising and people are getting put in harm's way as a result yeah I think that's the Royal we I read a book it says south African and you'll have to add it to the link So to
MIC1:Sure Yeah you
David:It's called how a Fox decodes the world basically it's scenario planning So when I talk about the Royal wheat it allows us to scenario plan and to figure out proactively what could happen if I move this liver and this lever happened what could actually happen And obviously we'll talk about the guy's name in a later date he actually got called by the FBI or the CIA cuz he predicted the world trade He basically saying look we've got disturbances over here got disturbances over there We've got this uprising going on Something is going to happen And we believe it's gonna happen in New York And he predicted that before the event happened So after it happened he got a knock on the door
MIC1:How did you know
David:did you know it's scenario planning It's forecasting is what he's is what he's talking about So is that not predict Maintenance Is that not predictive scenarios So if I have the data then I should be able to figure out how I can fix things proactively by being able to apply the right levers to get a better solution a better answer or going back to that train if I'd have made sure if I'd have taken care of some of those leaders early Right then I can predict when I should be changing those assets reduce my overall burden reduce my cost eliminate the risk of the organization and potentially eliminate the risk of having a rail derailment or something like that
MIC1:so to close it's close this chapter we've talked all
David:too much
MIC1:I don't know we've talked about the people problems the business challenges the even some of the theoretical whys So let's land this plane And just talk about some of the things that we're doing from a technology perspective What are some examples Some like concrete examples of the types of technology that we have access to today that we're using to pull this disparate data together into insight
David:Yeah So I think technology is finally caught up let me even give you an example of a few years ago GE pred predicts There was there was a whole lot of effort going on and they were gonna define the architecture and stitch the magic solution together Make a long story short There was a lot of people that lost their jobs I think the CEO of that department lost his job GE is no longer the fi fortune 500 company that it was And that's because they were they struggled with the architecture to stitch it all together The intent was good Right What they wanted to do was fantastic but I don't think the technology was ready I think now we're at an IOT conference I think the ability to to pull Infor information on demand in real time is pretty much there I think the ability to connect to that interoperably like an iPhone with APIs and GOs it's What we need to work on now is what is the actual solution and where are we pulling that information from That's all
MIC1:I'm hearing data Liquidity
David:Yeah data is quite possibly worth more than the assets the physical assets right but what is missing it's the content We now have the contextual agent called software technology the ability to pull stuff in but the content is what is missing We need to harness that content We need to digitalize that to Futureproof your organization other organizations and then potentially to to Futureproof our place on this earth So we can actually solve some of these bigger problems cuz we're all connected
MIC1:I love that We talk about connectivity so often be like oh it's technology And in all of the conversations I've had at the show people use the word interchangeably of connectivity and connected is actually connecting people
David:technology should be the new fish It transcends language and it should create an image that we can all recognize that transcends all the different languages because most of us are visual learners I shouldn't say all but we can all move into a picture that we can see we can touch We can feel we can almost feel now to me that's where technology can transcend a lot of these geopolitical and language issues and diversity issues If we do it right we do
MIC1:Thank you I think we've got all the opportunity to help build that Babel fish and bring everything all together and solve some real problems
David:I hope so
MIC1:this is just a fascinating conversation because it's so people centric and I hate when we lose sight of that So thanks for your time Dave Appreciate it
David:You're welcome
Ryan:the oil oil T We're at the oil T I'm gonna say that over and over again right
David:T conference for oil and gas